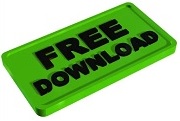


(For example, if n=100, and p=1/4, then the average number of successful results will be 25) If X ~ B( n, p), that is, X is a binomially distributed random variable, n being the total number of experiments and p the probability of each experiment yielding a successful result, then the expected value of X is: What is the probability of achieving 0, 1., 6 heads after six tosses? Mean Suppose a biased coin comes up heads with probability 0.3 when tossed. Some closed-form bounds for the cumulative distribution function are given below. It can also be represented in terms of the regularized incomplete beta function, as follows: the greatest integer less than or equal to k. The cumulative distribution function can be expressed as: Note that the probability of it occurring can be fairly small. M is the most probable ( most likely) outcome of the Bernoulli trials and is called the mode. In this case, there are two values for which ƒ is maximal: ( n + 1) p and ( n + 1) p − 1. Ƒ( k, n, p) is monotone increasing for k M, with the exception of the case where ( n + 1) p is an integer. There is always an integer M that satisfies This k value can be found by calculatingĪnd comparing it to 1. Looking at the expression ƒ( k, n, p) as a function of k, there is a k value that maximizes it. The probability mass function satisfies the following recurrence relation, for every : This is because for k > n/2, the probability can be calculated by its complement as In creating reference tables for binomial distribution probability, usually the table is filled in up to n/2 values. However, the k successes can occur anywhere among the n trials, and there are different ways of distributing k successes in a sequence of n trials. k successes occur with probability p k and n − k failures occur with probability (1 − p) n − k. The formula can be understood as follows. Is the binomial coefficient, hence the name of the distribution. The probability of getting exactly k successes in n trials is given by the probability mass function: In general, if the random variable X follows the binomial distribution with parameters n ∈ ℕ and p ∈, we write X ~ B( n, p). However, for N much larger than n, the binomial distribution remains a good approximation, and widely used. If the sampling is carried out without replacement, the draws are not independent and so the resulting distribution is a hypergeometric distribution, not a binomial one. The binomial distribution is frequently used to model the number of successes in a sample of size n drawn with replacement from a population of size N. The binomial distribution is the basis for the popular binomial test of statistical significance.
#Binomial distribution cdf trial#
In probability theory and statistics, the binomial distribution with parameters n and p is the discrete probability distribution of the number of successes in a sequence of n independent yes/no experiments, each of which yields success with probability p.Ī success/failure experiment is also called a Bernoulli experiment or Bernoulli trial when n = 1, the binomial distribution is a Bernoulli distribution.

The probability that a ball in a Galton box with 8 layers ( n = 8) ends up in the central bin ( k = 4) is.
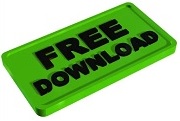